The Rise Of Reinforcement Learning: Shaping Our AI Driven Future
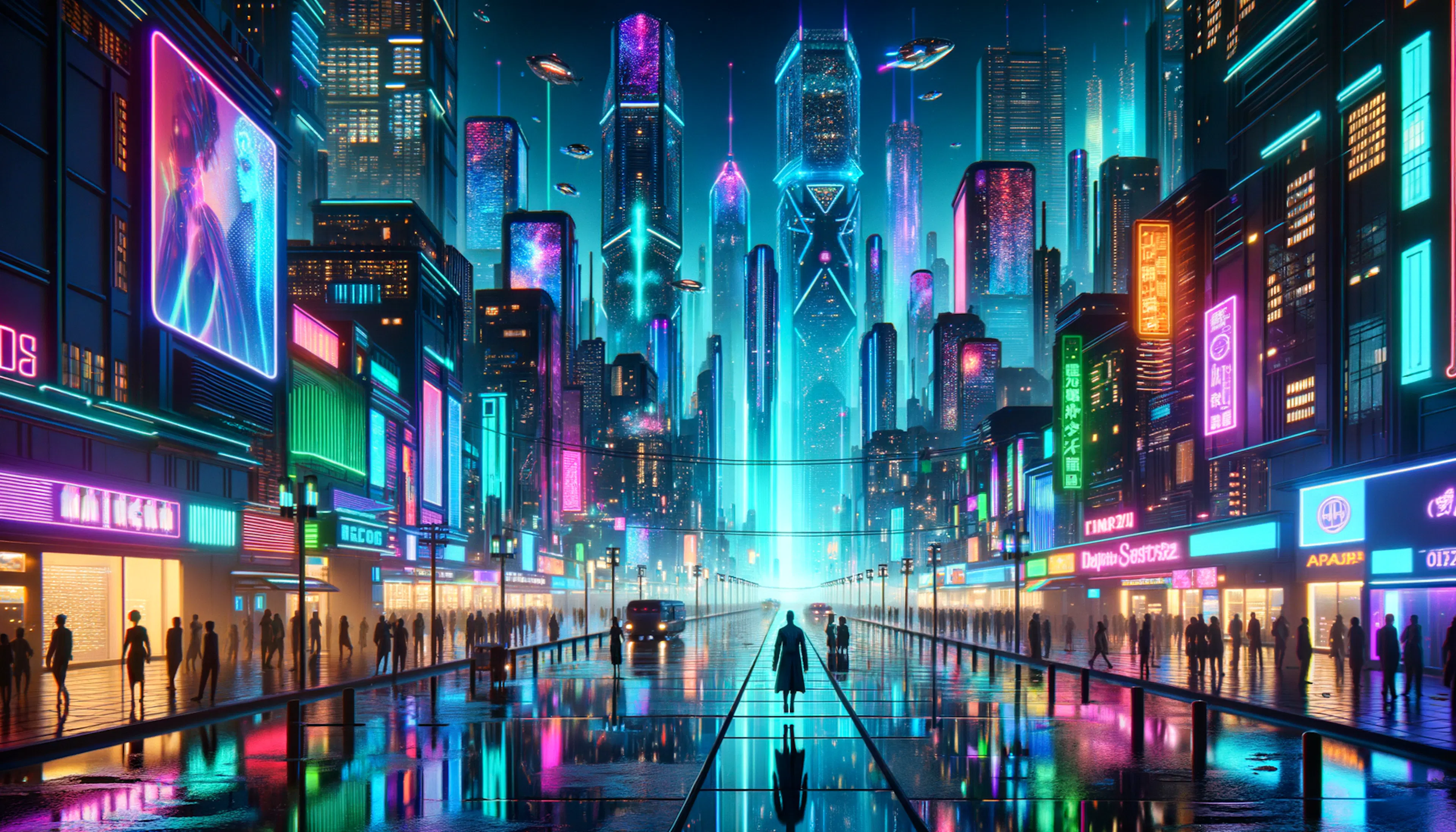
In the rapidly evolving landscape of artificial intelligence, reinforcement learning (RL) stands out as a powerful and promising approach. As we venture deeper into the 21st century, RL is poised to revolutionize how machines learn and interact with the world around us. This blog post explores the potential trajectory of reinforcement learning over the next decade and its far-reaching implications for society.
The Foundation of Future AI
Reinforcement learning, at its core, is about teaching machines to make decisions through trial and error. Unlike traditional machine learning methods, RL allows AI systems to learn from their own experiences, much like humans do. This approach has already shown remarkable success in various domains, from game-playing AI to robotics.
Over the next decade, we can expect RL to evolve in several key areas:
1. Enhanced Generalization and Transfer Learning
One of the most significant challenges in current RL systems is their ability to generalize knowledge across different tasks. In the coming years, we're likely to see substantial progress in this area:
- Meta-learning algorithms will enable RL agents to learn how to learn, adapting quickly to new environments and tasks.
- Transfer learning techniques will allow knowledge gained in one domain to be applied effectively to others.
- RL systems will become more versatile, capable of handling a wider range of real-world scenarios with minimal retraining.
This enhanced generalization capability will make RL systems more practical for deployment in complex, dynamic environments such as autonomous vehicles, smart cities, and adaptive industrial processes.
2. Integration with Other AI Technologies
The future of RL lies not in isolation but in its synergistic combination with other AI technologies:
- Natural Language Processing (NLP): RL agents will become better at understanding and generating human language, leading to more intuitive human-AI interactions.
- Computer Vision: Integration with advanced visual processing will allow RL systems to better perceive and interact with the physical world.
- Quantum Computing: As quantum computers become more accessible, they may dramatically accelerate RL algorithms, solving previously intractable problems.
"The fusion of reinforcement learning with other AI disciplines will create a new generation of intelligent systems capable of tackling some of humanity's most pressing challenges."
3. Ethical AI and Explainable RL
As RL systems become more prevalent in decision-making processes, there will be an increased focus on making these systems ethical and transparent:
- Development of interpretable RL models that can explain their decision-making process in human-understandable terms.
- Implementation of
fairness constraints
to ensure RL systems do not perpetuate or amplify societal biases. - Creation of robust safety mechanisms to prevent RL agents from taking harmful actions, especially in critical applications.
4. Real-world Applications and Societal Impact
The next decade will likely see RL moving beyond research labs and niche applications to become a ubiquitous part of our daily lives:
- Healthcare: RL systems could optimize treatment plans, drug discovery processes, and personalized medicine.
- Finance: Advanced RL algorithms may revolutionize trading strategies and risk management in financial markets.
- Education: Personalized learning experiences tailored by RL agents could transform how we acquire knowledge and skills.
- Environmental Conservation: RL could optimize resource management and conservation efforts, helping address climate change challenges.
Conclusion: Navigating the RL-Driven Future
As reinforcement learning continues to advance, it promises to bring about transformative changes in various aspects of our lives. From more efficient and personalized services to groundbreaking scientific discoveries, the potential benefits are immense. However, this progress also comes with responsibilities and challenges.
We must remain vigilant about the ethical implications of increasingly autonomous AI systems. Ensuring that RL technologies are developed and deployed responsibly will be crucial to harnessing their full potential while mitigating risks.
As we stand on the brink of this RL revolution, one question remains: How will we, as individuals and as a society, adapt to a world where AI systems are not just tools, but active decision-makers shaping our environment?